Other recent blogs
Predictive Analytics is powerful. It tells you about the future.
Almost every business and industry is increasingly investing in these prediction models and technologies to gain a clearer picture of the future and use the generated insights to tame risks and capture new opportunities. The Oil & Gas industry is no exception.
This blog is essential for business and technology teams operating in the oil and gas industry who are still on the fence about the impact of Predictive Analytics on their workflows and bottom lines.
In this blog, you will learn about the fundamentals of Predictive Analytics, how it works, and how it helps oil and gas companies.
What is Predictive Analytics?
Predictive Analytics is the practice of diving deep into massive datasets and finding patterns and trends that tell something about an impending threat or opportunity.
It’s a form of advanced analytics that thrives with the help of new and emerging technologies, such as AI, ML, Big Data, etc.
Data teams make use of historical data to train Machine Learning algorithms to predict future outcomes and unearth valuable findings to empower decision-makers across roles and enterprises.
How does Predictive Analytics work?
Predictive Analytics models are developed using larger, broader pools of historical and current data. And companies across industries, including utilities, retail, banking and financial services, and manufacturing, are using Predictive Analytics capabilities to streamline their operations, expand customer base and business revenue, and accelerate innovation.
The tools and technologies might be different from one data team to another depending on the problem to be solved and the nature of the datasets; however, the workflow almost always remains the same:
- Determine the business problem to be solved: This is the first step in the Predictive Analytics model development. Data scientists first need to understand the business problem that the model will likely solve; whether the organization is struggling with excessive machine downtime or needs help with deciding on the optimal inventory levels, it is imperative to first identify the challenge that potential Machine Learning models are expected to solve for the organization.
- Identify data sources and organize datasets in repositories like BigQuery: Developing highly sustainable and accurate predictive models requires troves of neat and clean data. To make this happen, the Data team must analyze its current data environment and sources through which it acquires data - it could be decades of data stored by the organization or a continual flow of customer data coming from various social sites and customer touch points.
Once the team identifies the data sources and the data sets that need to be collected and analyzed, the next step is to store that data in appropriate data repositories such as data warehouses. AWS, Google Cloud, Microsoft, and many others in the realm of Cloud Computing provide highly robust, secure, and affordable storage solutions. - Clean the data: Raw data does not hold much value for business use. To generate truly transformative insights that impact every aspect of your business, your data must go through a series of steps so it can be turned into ‘gold for your business.’
Fortunately, Data teams can access plenty of tools and platforms to clean troves of raw data in a seamless and affordable way. Some industry-leading tools and platforms in this space include OpenRefine, Drake, Winpure, Cloudingo, and TIBCO Clarity. - Build the predictive models: This is the fourth step in the workflow and involves the actual work of developing safe, secure, and highly reliable predictive models that can serve the intended purpose of the organization.
Data scientists can leverage a diverse set of tools and platforms to develop predictive models based on the business need and the nature of the datasets. Typically, regression models, machine learning models, and decision trees form most of the predictive models. - Validate and launch: It’s the final stage in the predictive model development workflow. Herein, the team needs to validate the predictions/results of the models and keep tweaking the models to achieve improved results. Once the models provide satisfactory results, the data team can make the models available to the decision-makers across the organization.
Predictive Analytics for oil and gas companies (5 ways it stands to transform the energy market)
Predictive Analytics - an organizational ability to look into the future with the help of reliable data - is a powerful tool that winners across industries are leveraging to gain increased visibility into their operations and drive business decisions. In the energy landscape, it is helping businesses across all three levels - upstream, midstream, and downstream.
Predictive Analytics: Unlocking new value for the oil and gas companies
Predictive Analytics capabilities can help companies across upstream, midstream, and downstream segments in the following ways:
- Reduced unplanned downtime: The O&G operations are driven by numerous critical and high-value assets. Unplanned downtime of machines and equipment can cost a company a fortune.
By harnessing the volatility around machine operations, operators can significantly impact the company’s bottom line and improve business performance and revenue. Powered by critical real-time data and insights, decision-makers can predict machine failures and maintenance needs and, thus, significantly reduce the fears and uncertainties surrounding unexpected machine failures. - Improved equipment reliability and availability: Operators and business leaders gain more visibility into on-shore and off-shore operations using Predictive Analytics reports and insights.
Data and insights on the health and performance of mission-critical assets help improve their reliability and availability. This new capability, in turn, improves an operator’s ability to capture more value from machines and assets and impacts the bottom-line performance. - Increased business revenue: Streamlined and Data-driven operations and decisions enable a company to successfully reduce its operational costs and significantly improve business revenue.
The strategic deployment of digital technologies, Predictive Analytics being one major component of the entire tech stack, can transform an O&G company’s operations and help the team regain control over its assets. - Capture new opportunities: Staying ahead of the competition requires a forward-looking strategy. Predictive Analytics helps these companies get a glimpse into the future, identify emerging trends hidden in the data sets, and rapidly respond to those trends/customer demands.
Oil and gas is a very capital-intensive industry, and being able to access even the slightest degree of capability to predict future outcomes can help steer the organization toward new growth frontiers.
A few final thoughts
There is no denying the fact that embedding Predictive Analytics within decades-old processes is not going to be an easy fit for most organizations.
However, with the right mindset and compatible tools and technologies, you can build Predictive Analytics within your systems and processes. You can accelerate the journey by engaging a trusted Predictive Analytics partner like Kellton.
At Kellton, we have our in-house comprehensive offering for the oil and gas companies. We call it ‘Optima.’ It is an IoT-enabled digital oilfield analytics platform for seamless operations and resource management.
Over the years, we have helped businesses of all shapes and sizes across the O&G industry with their data challenges and build end-to-end ecosystems to harness the true potential of their data. To kickstart your journey towards a more robust and scalable Data Analytics ecosystem, connect with us here.
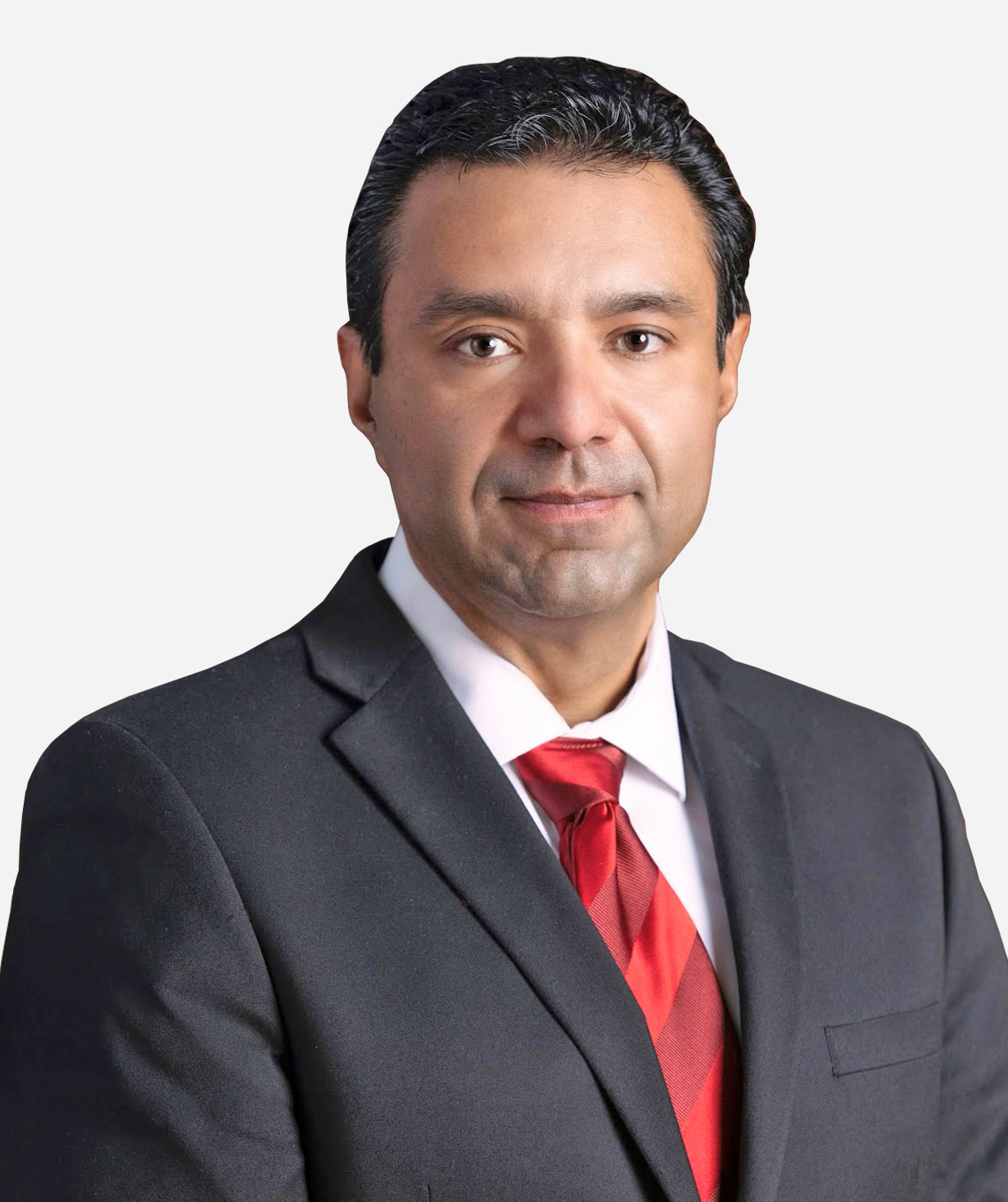